Towards an Accountability Framework for AI Systems: The Autonomous Vehicle Use Case
Contact: Rainer Trauth, M. Sc.
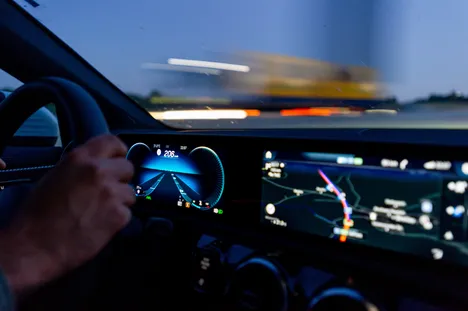
The progress in the field of autonomous driving is tremendous. New technologies through the development of artificial intelligence are making this progress possible. Today, the first autonomous vehicles are already driving thousands of kilometers on test routes without the need for major intervention of human drivers. Developments in this area are accompanied by increasingly powerful algorithms and methods from the field of machine learning. However, the increasing complexity of the used techniques also creates a more opaque environment in terms of decisions made by the technological tools. This lack of transparency means that certain decisions made by the vehicle are neither recognizable nor understandable to the user, the developer or the legislator.
AI’s non-transparent behaviour is usually referred to as a “black-box,” meaning that only the input and output variables are known to the developer. Explainable AI methods are concerned with resolving precisely this opacity and making complex AI systems more understandable and interpretable. This often conflicts with the fact that developers and researchers are searching for quick solutions to technical problems, leaving the questions of transparency and accountability on the sidelines.
However, transparency is necessary for a broad market introduction of autonomous vehicle systems, as it is the basis of trust and the effective implementation of legislation. The aim of the research project is to develop an accountability framework in the field of autonomous driving, taking various stakeholder interests into account. To work towards the overall research goal of how explainable AI can solve issues of accountability in autonomous driving, three main questions will be investigated:
(1) How can explainability impact/improve acceptability and usability for the user (internal, external, technical)
(2) How can responsibility be distributed based on the implementation / integration of explainability?
(3) Based on the user requirements, how can we integrate explainability in the AVs system?
The research project will play a fundamental role in developing new approaches and designing comprehensive tools and frameworks to help navigate these issues and find reasonable and defensible answers.
Researchers:
- Auxane Boch, Institute for Ethics in AI, TUM
- Ellen Hohma, Institute for Ethics in AI, TUM
- Rainer Trauth, Institute of Automotive Technology, TUM