Titel | BA | SA | MA | HW | exp | the | kon | Eintrag |
---|---|---|---|---|---|---|---|---|
Task Force - Real-World Autonomous Driving: Object Tracking and Motion Prediction in Urban and Racing Application | 2025-02-05 | |||||||
Trajectory Enhanced Object Detection with Transformers for Autonomous Driving | 2025-02-05 | |||||||
Motion Prediction Development for Urban and Racing Autonomous Driving Applications - IDP | 2025-02-05 |
Loïc Stratil, M.Sc.
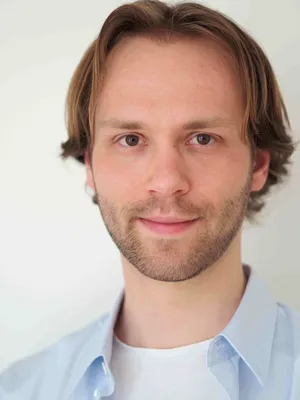
loic.stratil(at)tum.de | |
Room | MW 3508 |
Phone | +49.89.289.15898 |
Fax | +49.89.289.15357 |
Research
Autonomous driving (AD) technology is advancing rapidly. However, for open market use, its software must be highly reliable—particularly regarding how the vehicle perceives its environment both in the present moment and in anticipating future changes. Traditionally, AD perception relies on sequential Detection, Tracking, and Prediction (DTP) modules. Recent research shows that systems can perform better when these modules share information dynamically rather than working in isolation. Such integrated approaches are critical for achieving the precision and adaptability needed in real-world driving conditions.
My research, therefore, focuses on developing DTP architectures capable of understanding the vehicle’s environment in depth—both physically and semantically. This rich contextual knowledge enables more accurate predictions of other agents' long-term movements. I explore both sequential information-sharing methods and end-to-end architectures to maximize the adaptability and precision of AD perception systems in real-world applications.
Keywords: Perception 2.0, Detection - Tracking - Prediction, End-to-End Perception, Scene Understanding, Deep Learning, EDGAR